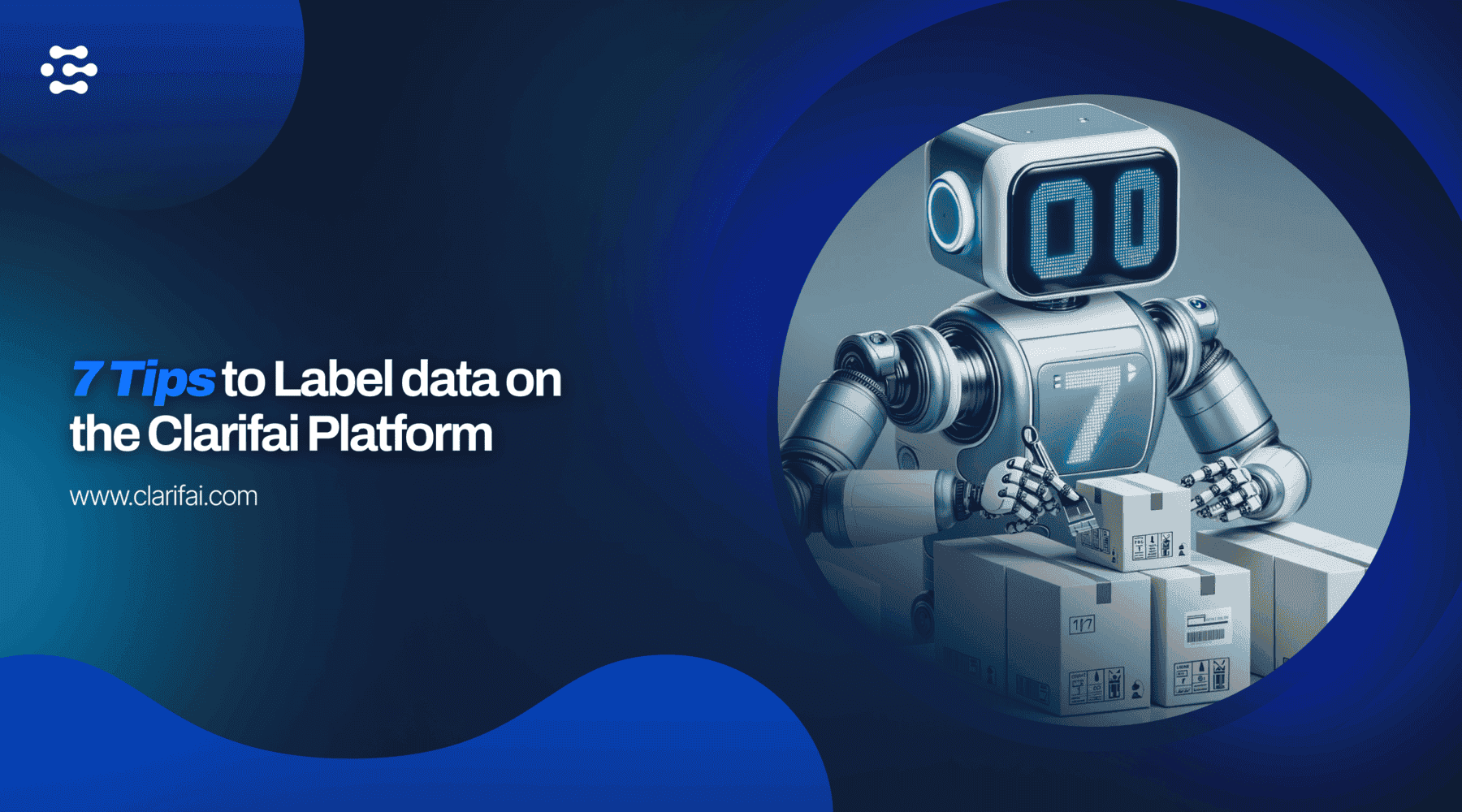
While building a machine learning project, managing and labeling the data play a key role. The quality of input data directly impacts the model's performance, making data labeling a crucial stage in training the Machine Learning models.
At Clarifai, we’re constantly releasing new features that help AI developers optimize their training data production throughput, efficiency, and quality. In this blog, let's explore 7 extremely useful tips for labeling your data using the Clarifai Platform.
Let's start with the 3 frameworks that Clarifai offers for getting your inputs labeled, each with their own specific benefits.
1. Labeler Tool
The Labeler tool allows you to work as a team, sharing the workload with collaborators and offers a streamlined no distractions view to manage labeling tasks that helps maintain focus and efficiency.
You can also view all related tasks, activities and statistics for your application.
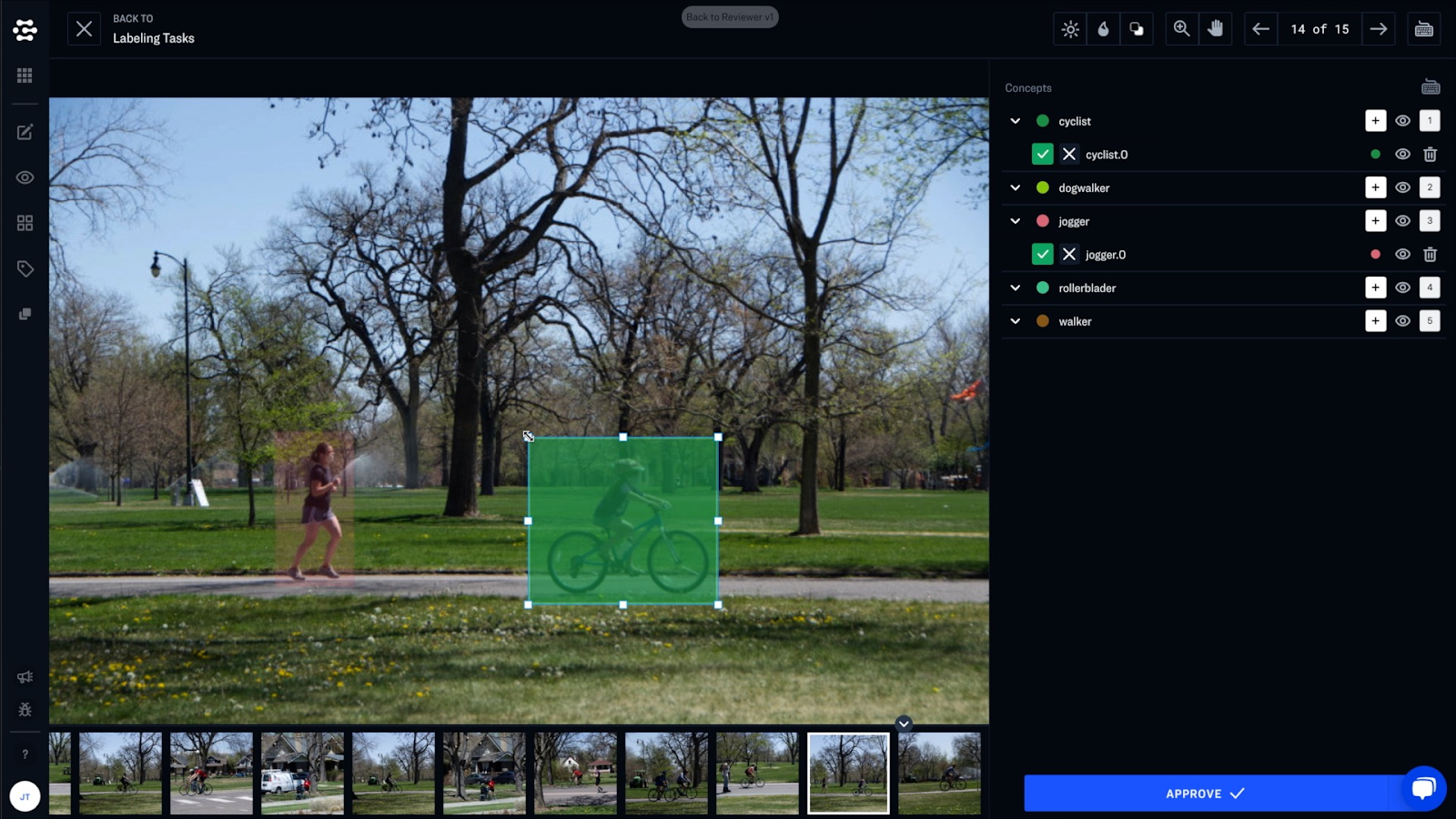
2. Portal’s Grid View
Using the Portal's Grid View for labeling inputs can speed up your modeling process. By using the Input Upload button, you can quickly add labels in bulk to selected inputs right from the moment they are chosen for upload. Additionally, you can include them in datasets to start training models.
Furthermore, you can add metadata in bulk, providing three different levels of image retrieval and categorization all from one screen.
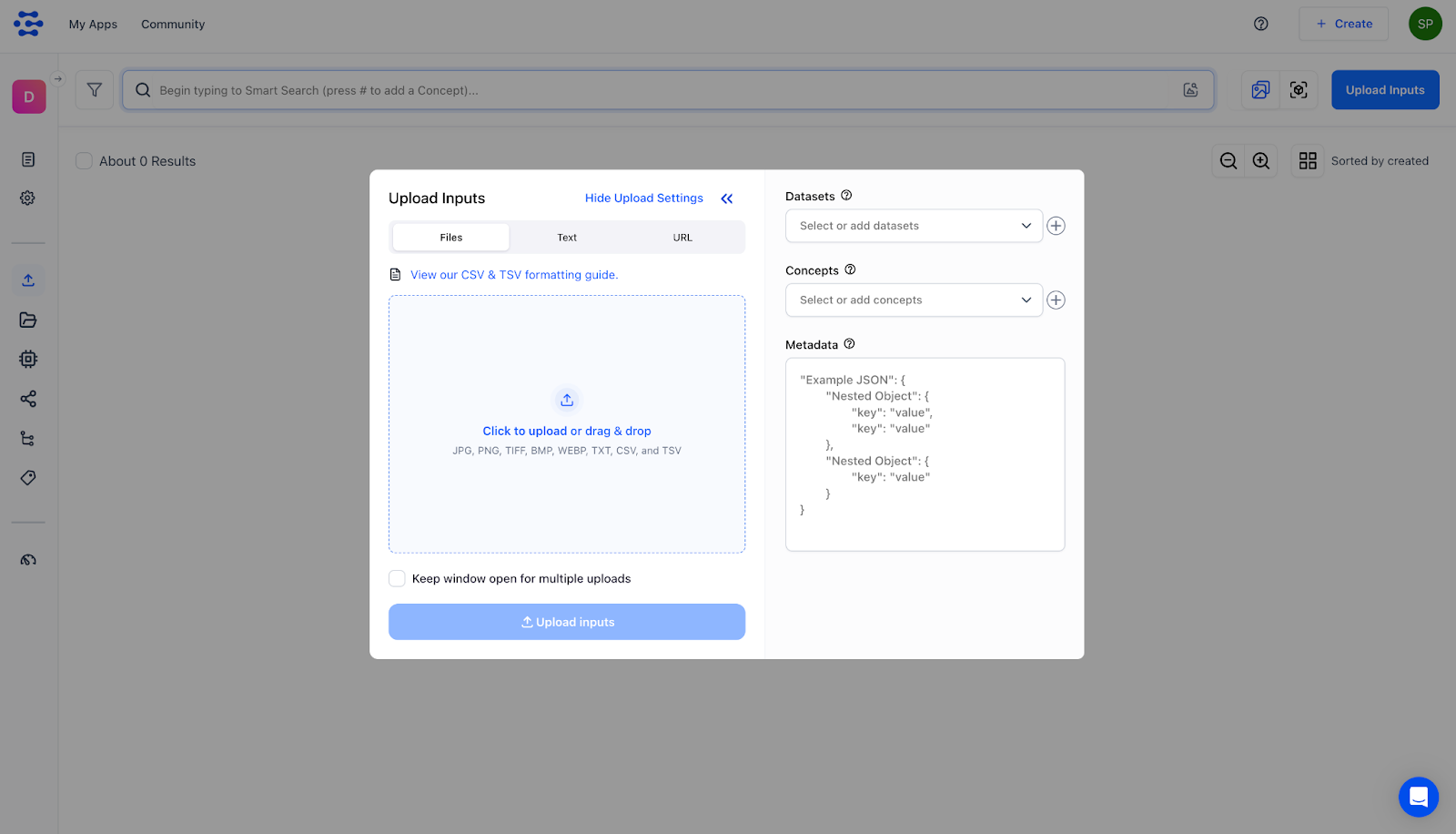
3. Portal’s Input Viewer
When accuracy and specific details are essential, turn to the Portal's Input Viewer. Accessing the full range of Clarifai's labeling tools like Bounding Box, Polygon, Select/Edit and others for each individual input. This allows maximum flexibility while working. You can also add specific input metadata from this screen.
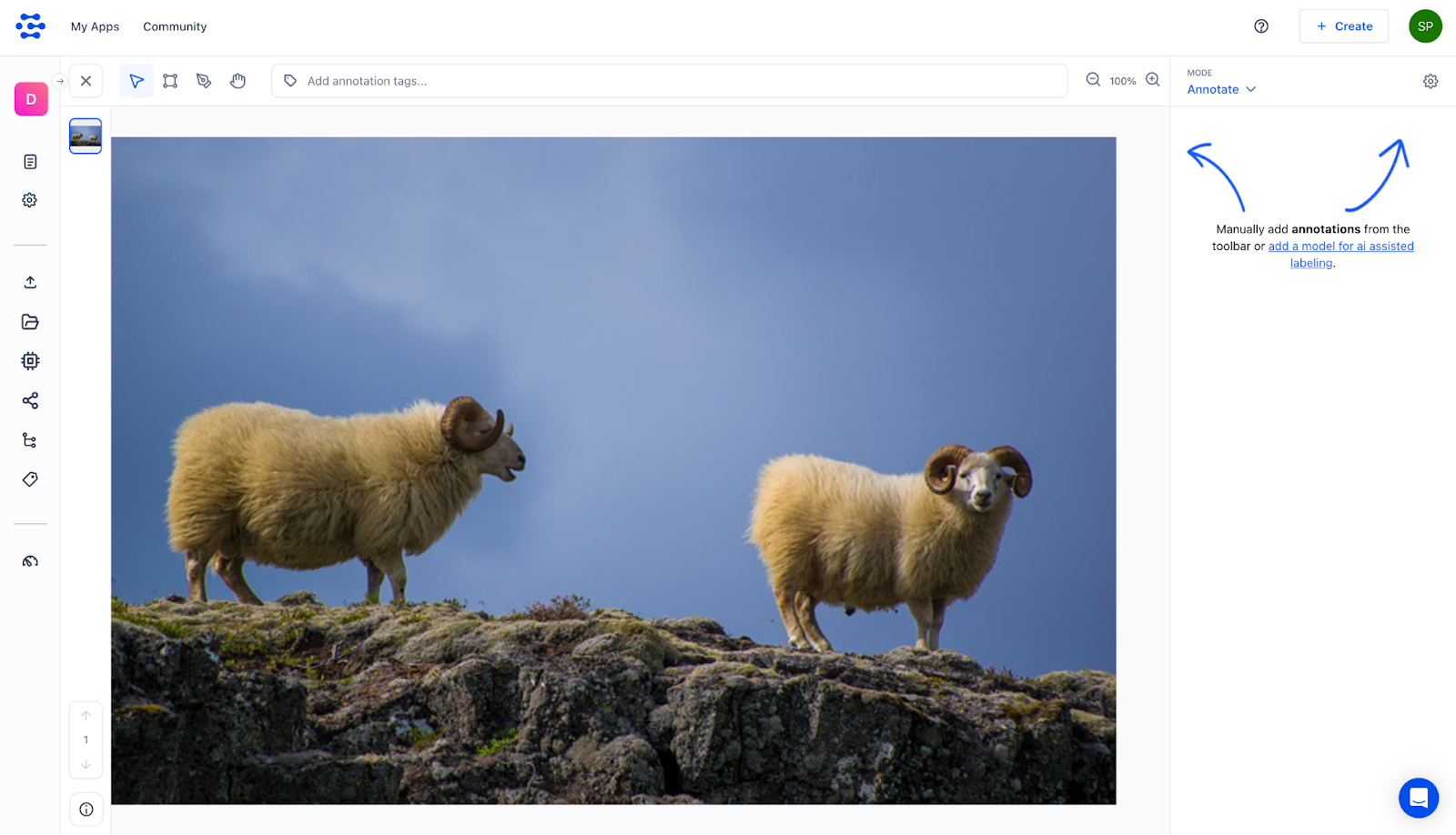
We also provide AI tools that significantly accelerate your workflow on top of these tool frameworks. Let's look at them!
4. AI Assist
The AI Assist feature lets you put the power of Clarifai's models to work immediately. While using either Labeler or Input Viewer, you can use a pre-existing model to suggest concepts that are present in your input simply by creating a workflow before you begin labeling.
This can be helpful in identifying complex instances of concepts or boosting efficiency, enabling you to directly jump to the review phase of labeling.
Check out this Video to learn more about AI Assisted Labeling!
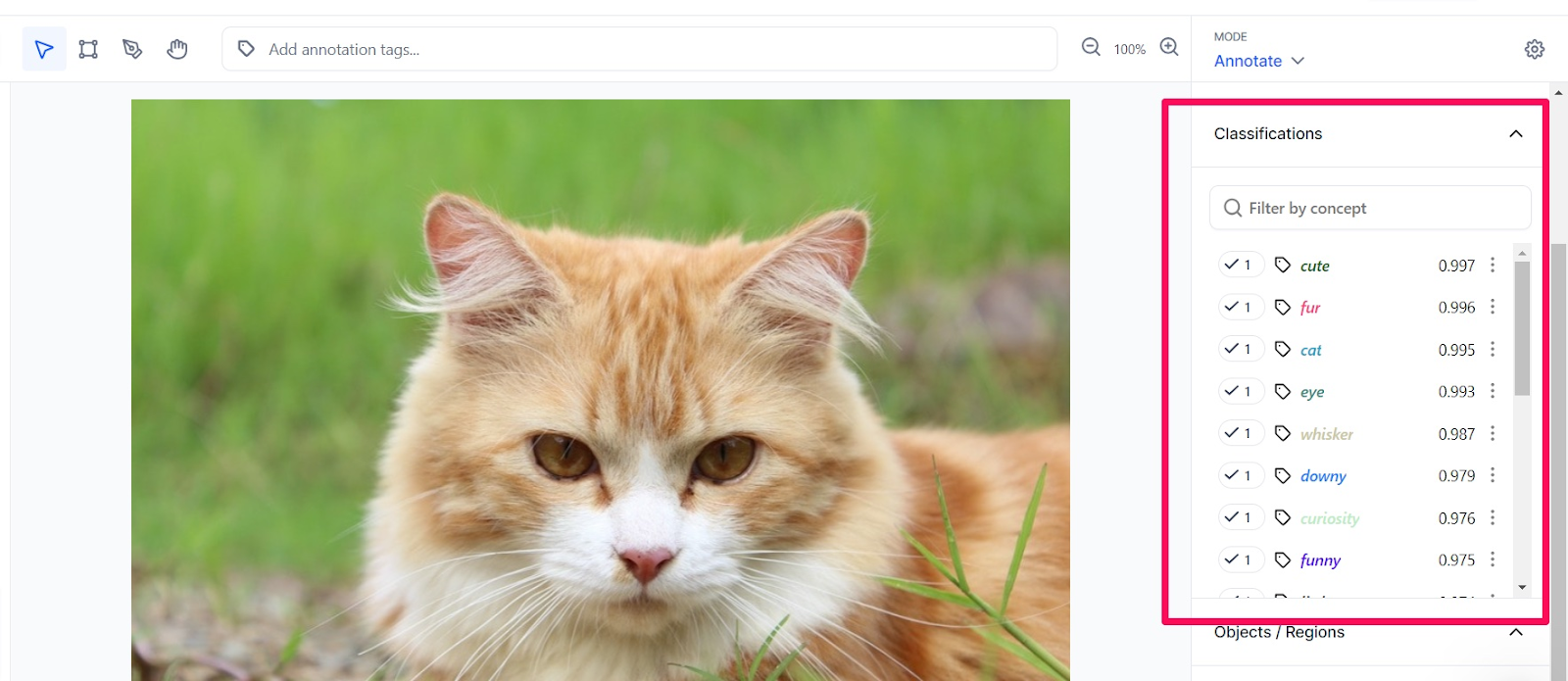
5. Auto Annotation
Maximizing the potential of AI Assist, the Auto Annotation feature enables you to label inputs using a pre-existing model or workflow, eliminating additional manual work. This feature is essential for continuously improving models and is supported within Clarifai's AI Lake, enabling continual labeling, training, and retraining using other features like Collectors and Workflows.
Checkout the guide on how to use the Auto Annotation feature here:
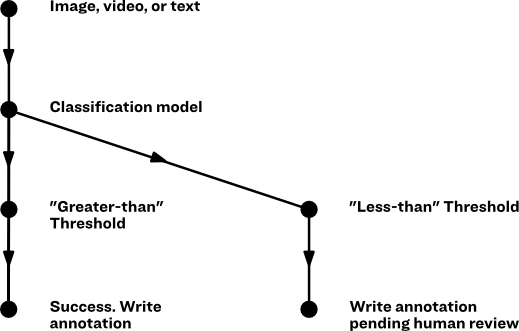
6. Visual Search
Using Clarifai's powerful Visual Search capabilities can significantly simplify tasks, whether you're searching for a specific item or identifying a concept based on visual recognition.
You can access this feature in the Portal Grid View, where searching for one input using visual search will return similar inputs with decreasing similarity based on visual cues and features.
This can be very useful when dealing with large amounts of unlabeled data. Additionally, when combined with the labeling feature in GridView, it becomes a very powerful part of your labeling toolkit.
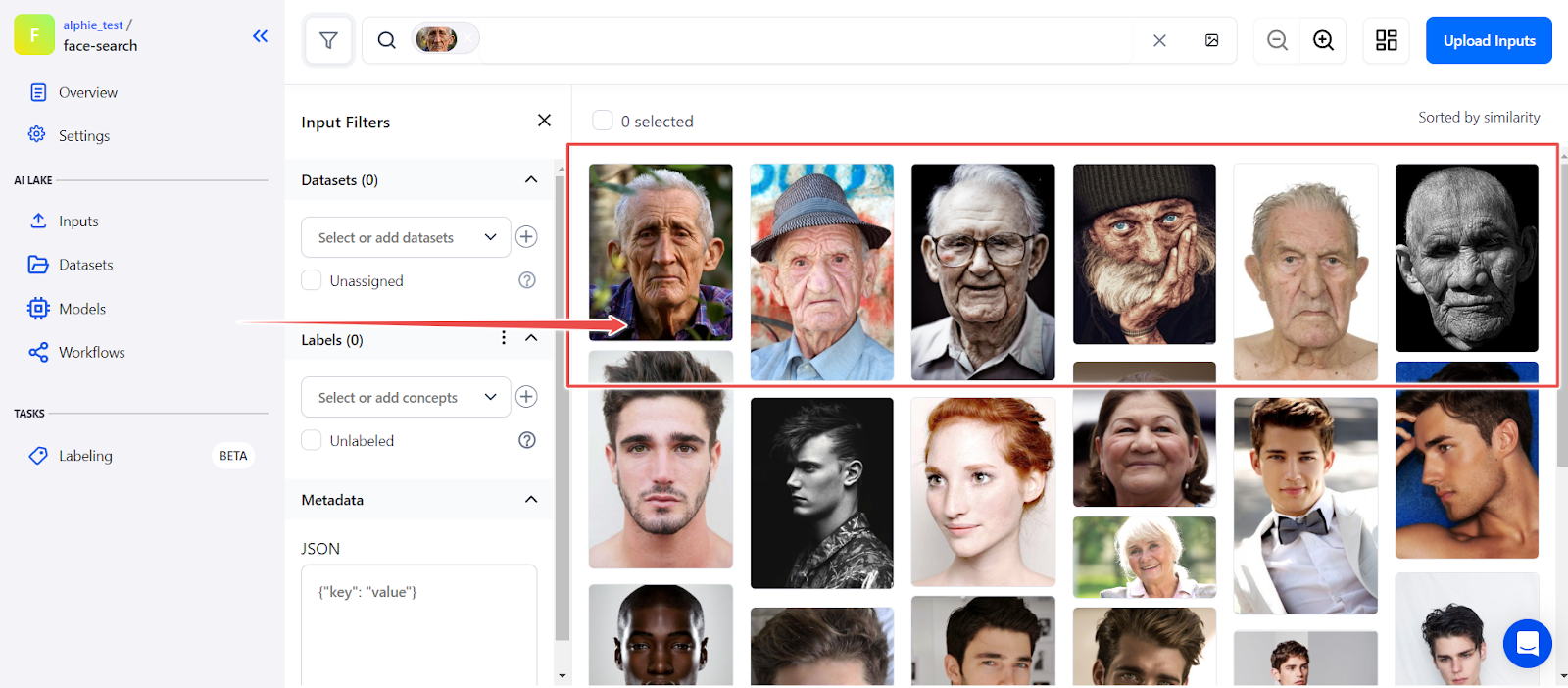
7. Smart Caption Search
Our latest feature, Smart Caption Search, lets you rank, sort, and retrieve images based on text queries.
By merging various semantic and embedding search capabilities, Smart Caption Search transforms your human-generated sentences or thoughts into powerful search queries across your inputs. Simply input a descriptive text that best describes the images you want to search for, and the most relevant matches associated with that query will be displayed.
Performing searches using full texts allow you to provide a much more in-depth context and retrieve more relevant results as compared to other types of searches.
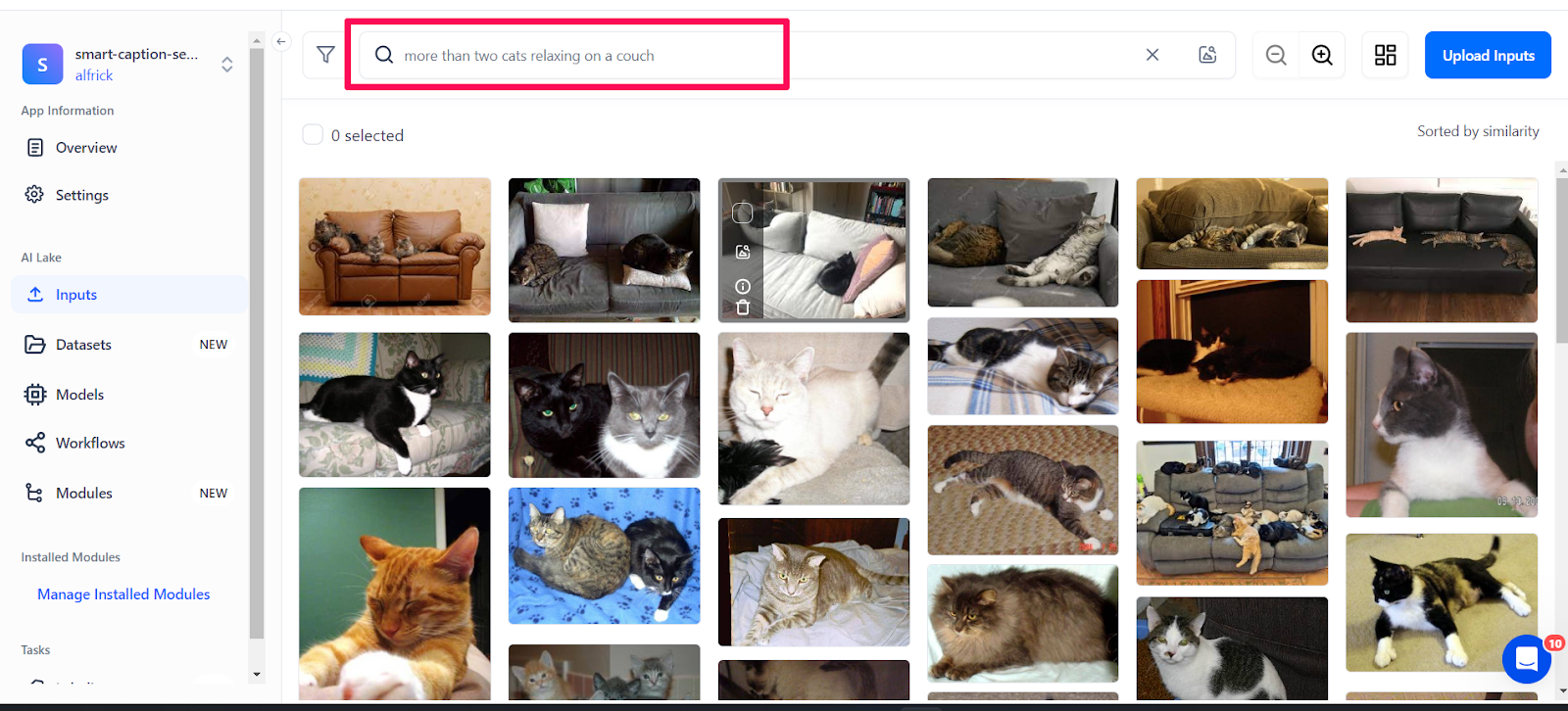
These techniques help you effectively label the data by making labeling more precise and accurate, encouraging teamwork, and speeding up model training.