The Future of Content Moderation: How Multimodal Moderation is Changing the Game
Introduction
Content moderation is an essential aspect of many online platforms, from social media to e-commerce sites. However, as the volume and variety of user-generated content continues to grow, traditional moderation approaches can no longer keep up. This is where multimodal moderation comes into play. Multimodal moderation is a revolutionary approach that combines different modalities such as text, image, video and audio to analyze and moderate content. By leveraging AI and human expertise, multimodal moderation can overcome the limitations of traditional moderation and provide a more accurate and efficient moderation solution.
In this blog post, we explore the benefits of multimodal moderation and offer insights from industry experts, tips, and strategies to help you gain a deeper understanding of this approach. We also review recent research from the University of Toronto in the area of multimodal moderation and explain how the company's content moderation capabilities incorporate the latest research. Whether you moderate content, run a platform, or are simply interested in the topic, this guide will provide you with the information you need to effectively implement multimodal moderation on your platform. Let's dive in!
What is Multimodal Moderation?
Content moderation has traditionally relied on text-based moderation, with algorithms or human moderators analyzing written content. However, with the advent of multimedia platforms, the need for a more sophisticated approach has become apparent. Multimodal moderation is a new approach that addresses this need by integrating different modalities for content analysis and moderation.
Multimodal moderation goes beyond text-based moderation to include analysis of visual, audio, and video content. This approach can better identify harmful or inappropriate content by analyzing contextual cues across multiple media formats.
Multimodal moderation can be achieved by using various artificial intelligence (AI) techniques such as natural language processing (NLP), computer vision, and speech-to-text. By combining these techniques, multimodal moderation can detect subtle nuances and nonverbal cues that may not be apparent in text-based moderation.
Human expertise also plays an important role in multimodal moderation. While AI can identify and flag potentially harmful content, human moderators can provide additional context and cultural knowledge that algorithms may lack. A combination of AI and human expertise can provide a more accurate and effective moderation solution.
Multimodal facilitation has many advantages over traditional facilitation methods. In the following sections, we'll learn about the importance of multimodal moderation and how it can help overcome the limitations of traditional moderation methods.
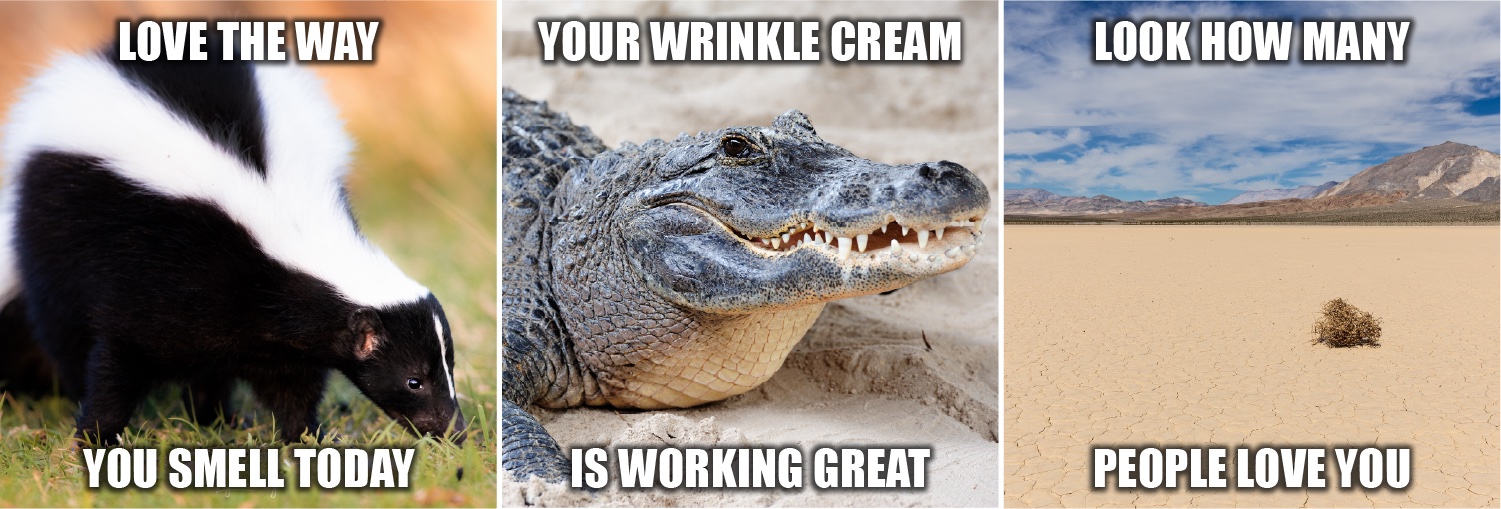
The Hateful Memes dataset contains real hate speech. So instead of showing actual memes from the dataset, we are instead showing only merely mean examples here. In each of these sample memes, the text phrase and the image are innocuous when considered by themselves. The semantic content of the meme becomes mean only when the text phrase and image are considered together.
Why is Multimodal Moderation Important?
Multimodal moderation is essential for online platforms to ensure the safety and well-being of their users. Here are some of the key reasons why multimodal moderation is important:
1) Multimodal moderation can uncover harmful content that may be missed with text-based moderation.
Many harmful behaviors and messages on the Internet are delivered through multimedia formats such as images, videos, and audio. These formats often contain subtle or implicit messages that are difficult to detect with text-based analysis alone. Multimodal moderation can detect these messages by analyzing multiple modalities simultaneously and provide a more comprehensive understanding of the content.
2) Multimodal moderation can reduce the risk of biased moderation.
Text-based moderation can be biased, especially when it comes to identifying harmful content that may be conveyed through cultural or linguistic differences. Multimodal moderation can provide a more nuanced understanding of content by analyzing multiple modalities, reducing the risk of biased moderation.
3) Multimodal facilitation can improve facilitation efficiency.
Traditional facilitation methods can be time and resource consuming. Multimodal moderation can help improve efficiency by automating certain tasks and enabling more accurate and comprehensive content analysis. This allows human moderators to focus on more complex tasks, such as analyzing context and providing additional information.
4) Multimodal moderation can adapt to new forms of content and behavior.
As online behavior and content continue to evolve, traditional moderation methods may become obsolete. Multimodal moderation is a flexible approach that can adapt to new forms of content and behavior by integrating different modalities and AI techniques.
In the following sections, we explore how multimodal moderation can overcome the limitations of traditional moderation methods and provide tips and strategies for implementing multimodal moderation on your platform.
Limitations of Traditional Moderation Methods
Text-based moderation is the common method for moderating content, but it has its limitations. Here are some of the main limitations of traditional moderation methods:
1) Limited analysis of non-textual content
Traditional moderation methods can only analyze textual content. This means that non-textual content such as images, videos, and audio, may be overlooked. Harmful messages can be conveyed through non-textual content, and without analyzing these modalities, traditional moderation methods may miss them.
2) Inability to recognize context
Text-based moderation can only analyze the content that is explicit in the text. The context or subliminal message that is implied cannot be detected. This makes it difficult to accurately detect harmful content hidden in a seemingly innocuous message.
3) Bias
Human moderators can be biased when analyzing content, especially when it comes to detecting harmful messages conveyed through cultural or language differences. Traditional moderation methods can also be biased due to the algorithms used to analyze the text.
4) Time-consuming and resource-intensive
Traditional moderation methods can be time-consuming and resource-intensive, especially for large platforms with a high volume of user-generated content. This can lead to delays in moderation, which can affect the safety and well-being of platform users.
Multimodal moderation can work around these limitations by analyzing different modalities simultaneously and providing a more comprehensive understanding of the content. In the next section, we'll explore how multimodal moderation can be implemented on your platform.
Implementing Multimodal Moderation on Your Platform
If you're interested in implementing multimodal moderation on your platform, here are some tips and strategies to get you started:
1) Identify the modalities to analyze
To implement multimodal moderation, you first need to identify the modalities to analyze. These can be text, images, videos, and audio. Depending on the platform and the type of content shared, you'll need to prioritize certain modalities over others.
2) Collect and label training data
To train your multimodal moderation model, you'll need a large dataset of labeled content. This can be a time-consuming process, but it's important to ensure the accuracy of your model. You'll also need to label content for different modalities such as text, images, and videos.
3) Train your model
Once you have your labeled dataset, you can begin training your multimodal moderation model. This can involve using machine learning algorithms to analyze the different modalities simultaneously and identify harmful content. It's important to refine and improve your model over time to ensure its accuracy and effectiveness.
4) Integrate with your platform
Once you've trained your model, you can integrate it with your platform's content moderation system. This may mean automating certain tasks, such as identifying potentially harmful content, and flagging it for review by a human moderator. You'll also need to provide guidelines and training for your human moderators to ensure they can use the multimodal moderation system effectively.
5) Monitor and refine
Multimodal facilitation isn't a one-time solution; it requires ongoing monitoring and refinement. You must continue to collect and label training data, train your model, and refine your moderation system to detect new forms of harmful content and behaviors.
Our content moderation capabilities can help you implement visual and text moderation on your platform. Our platform offers a suite of AI-powered tools to help you automate certain moderation tasks and improve the efficiency and accuracy of your moderation system. We also provide professional services and support to help you implement and refine your moderation system.
In the next section, we take a closer look at a recent study on multimodal moderation conducted by the University of Toronto.
Recent Research on Multimodal Moderation by the University of Toronto
We recently collaborated with the University of Toronto’s Division of Engineering Science on a 4th year capstone research project on multimodal moderation, which provides insights into the potential of this approach. The project focused on analyzing hateful memes on social media platforms, using a dataset of over 12,000 memes and their associated captions.
The student researchers used a multimodal approach to analyze the images and captions simultaneously and found that this approach outperformed text-based approaches in identifying hateful memes. By analyzing the visual and textual content together, the approach was able to detect implicit hateful messages that might have been missed with purely text-based approaches.
Specifically, one of the performant approaches utilized readily-available building blocks on our platform such as the CLIP image caption model to extract signals from the visual context.
Overall, the study provides compelling evidence for the effectiveness of multimodal moderation in detecting harmful content. By analyzing various modalities simultaneously, multimodal moderation can provide a more comprehensive understanding of content and help detect harmful messages that may be missed by traditional moderation methods.
If you're interested in implementing multimodal moderation on your platform, this study offers valuable insights into the potential of this approach. With our content moderation capabilities, you can implement multimodal moderation and improve the safety and well-being of your platform's users.
Conclusion
In summary, multimodal moderation is a promising approach to content moderation that can help better detect harmful content. By analyzing multiple modalities such as text, images, and videos, moderators can gain a more comprehensive understanding of content and identify harmful messages that may be missed by traditional moderation methods.
We discussed the benefits of multimodal moderation, including increased accuracy and a more holistic understanding of content. We also presented our own content moderation features.
If you're interested in implementing multimodal moderation on your platform, we encourage you to try out our content moderation capabilities. Our platform allows you to moderate content across multiple modalities, and our models are trained on vast amounts of data to ensure high accuracy.
Implementing multimodal moderation can help ensure a safer and more positive experience for your platform users, and we're here to support you every step of the way. Sign up for our platform today and see the benefits of multimodal moderation for yourself.
If you're interested in learning more about multimodal moderation and content moderation, there are a variety of resources available to help you deepen your understanding. Here are a few of our top picks: