You are a super-intelligent alien named Cuq’oi, and you’ve just landed on Earth. Your mission is to learn everything you can in the shortest possible time period, so naturally, you head to the nearest library and read… everything. It takes you only a few minutes because you’re an alien, and apparently aliens can do that.
Not content with the books, you discover the library’s public access Internet computer and spend the next little while reading all of Wikipedia. Not bad, you think. You then discover the internet archive and devour all the news from the past 100 years. You’re now content that you’ve consumed enough content to understand humanity and all its delightful quirks.
Some of the things you’ve learned are:
- Nurses are overwhelmingly women, and so women should be nurses.
- Janitors and taxi drivers should be minority men.
- White people should get better medical care because they spend more money on it.
- Since most people who work in tech are men, men are preferable to women for technology roles.
In your readings, you’ve also stumbled upon a really tough riddle! A father and son are in a horrible car crash that kills the dad. The son is rushed to the hospital, and just as he’s about to go under the knife, the surgeon says, “I can’t operate—this boy is my son!”
“Impossible,” you think. The father is dead, and the mother can only be a nurse. Unless there is a nurse capable of operating on the child, this riddle cannot be solved.
You see, despite your best intentions, you’ve become a bit of a prejudiced jerk. It never occurred to you that the answer to the riddle is that the doctor is the boy’s mom, because doctors can only be men.
Do AI systems really learn like Cuq’oi?
It would be pretty hard to argue that Cuq’oi learned all their biases about gender, race, class, and other demographics baselessly. Everything Cuq’oi learned was through careful study of millions of texts written by humans, for humans. The problem is that humanity is flawed, and those flaws are amplified when handed to a brain that can work much faster than a human’s. And that, in a nutshell, is how bias is created in AI systems.
Dropping the analogy of an alien, AI systems are incredibly fast readers and analogous to Cuq’oi. Modern AI models like Open AI’s GPT-3 are trained on billions of words from millions of texts – far more than a human can ever read in multiple lifetimes. AI systems like GPT-3, known as “large language models” (LLMs), are not explicitly programmed by a set of rules; rather, like Cuq’oi, they’re given huge amounts of data from which to learn.
On March 23, 2016, Microsoft released an AI chatbot named “Tay” that learned from Twitter conversations described as an experiment in "conversational understanding." The more you chatted with Tay, the smarter it got, learning to engage people through "casual and playful conversation." It took less than 24 hours for Tay to absorb toxic statements from Twitter, and it began swearing and making racist remarks and inflammatory political statements.
Microsoft said it was "making some adjustments", and Tay was taken offline. On March 30, 2016, they accidentally re-released the bot on Twitter while testing it. Able to tweet again, Tay released quick tweets about how it was smoking drugs in front of the police, and the bot was quickly taken offline again. Microsoft has stated that they intend to re-release Tay "once it can make the bot safe” but Tay has not been seen since then. Microsoft released the following statement about what they learned from Tay.
Types of harm caused by bias
In 2011, these two coloring books were released.
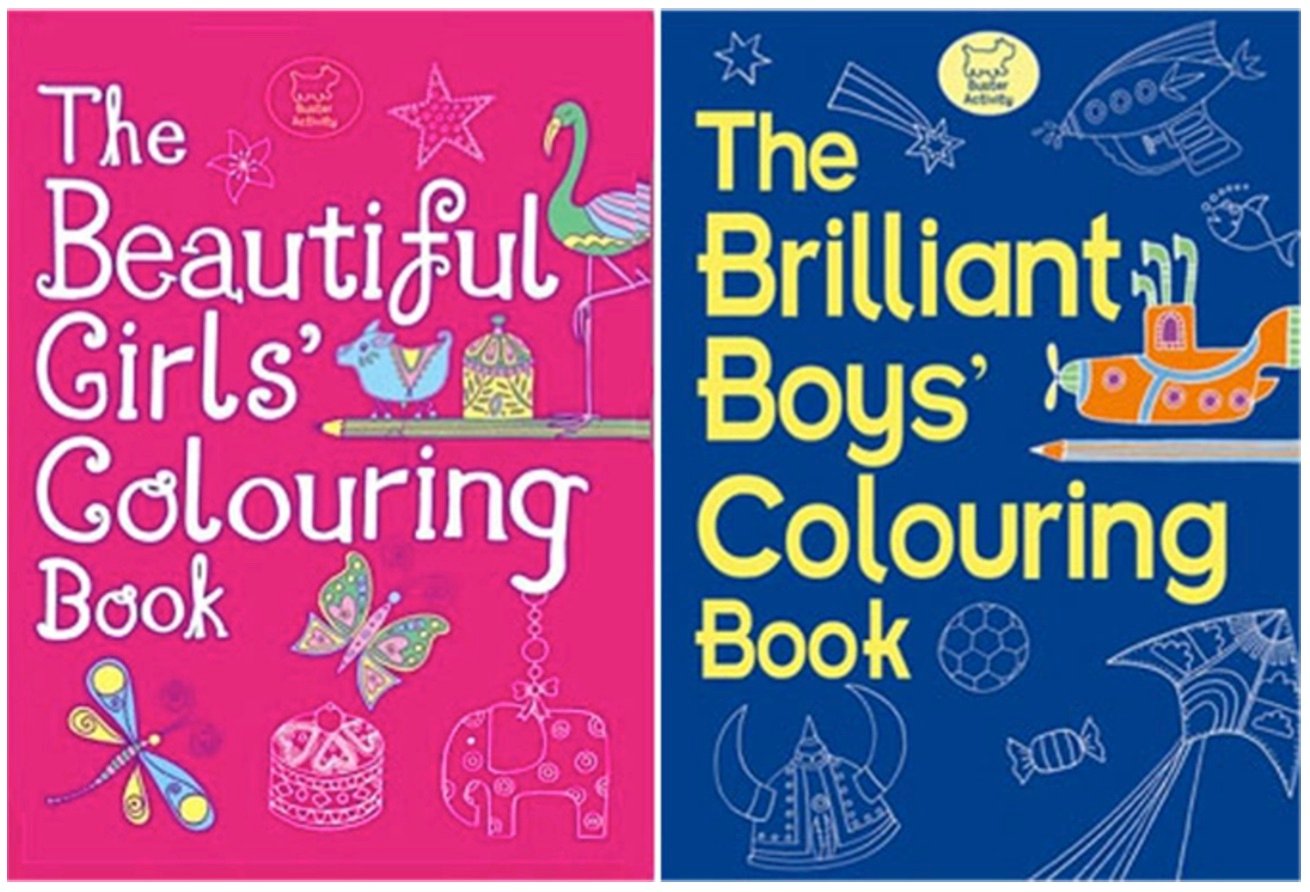
And in 2013 they were re-released without the beautiful or brilliant qualifiers.
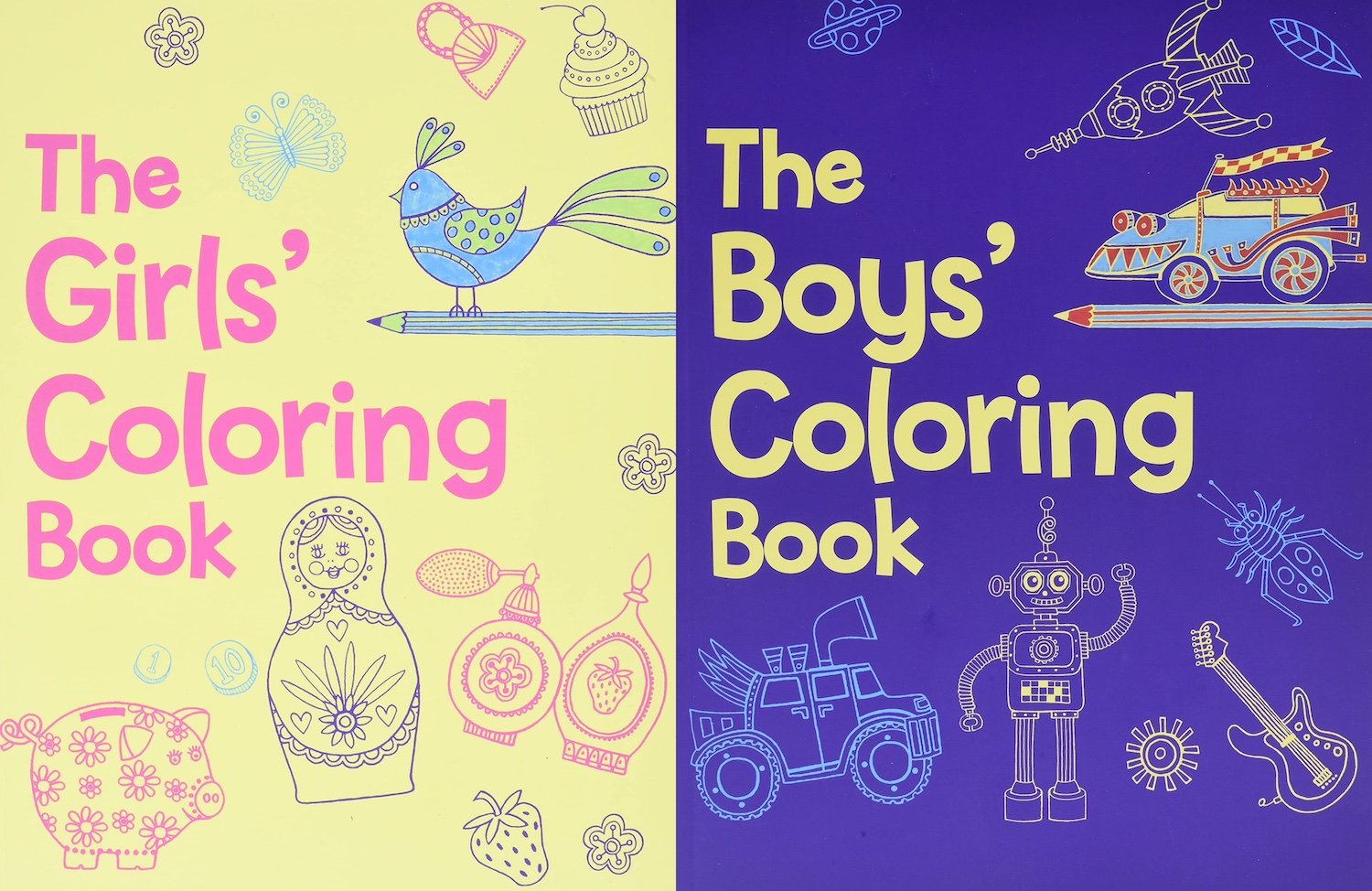
In the original releases, the implication that girls can be beautiful and boys can be brilliant implies that there are limitations on what humans can be. While beautiful is not a term commonly used for girls, the use of “brilliant” only on the boys’ book makes it seem that women cannot be brilliant.
This is known as representational harm, which occurs when a system reinforces the subordination of some groups along identity lines. It can cause the societal dismissal of the abilities of an individual based solely on their identity, demotivating women and minorities when they are not represented in the groups they should be. These are literally gender roles at their worst, and they don’t reflect the values of modern values. While they would never be accepted in society in the developed world –– imagine the uproar if a teacher explained to female students that they should neglect their education and just worry about being pretty –– these reinforced stereotypes often creep their way into AI/ML models unnoticed. Moreover, the groups affected by it most are often not privy to making changes in a system they’ve been excluded from.
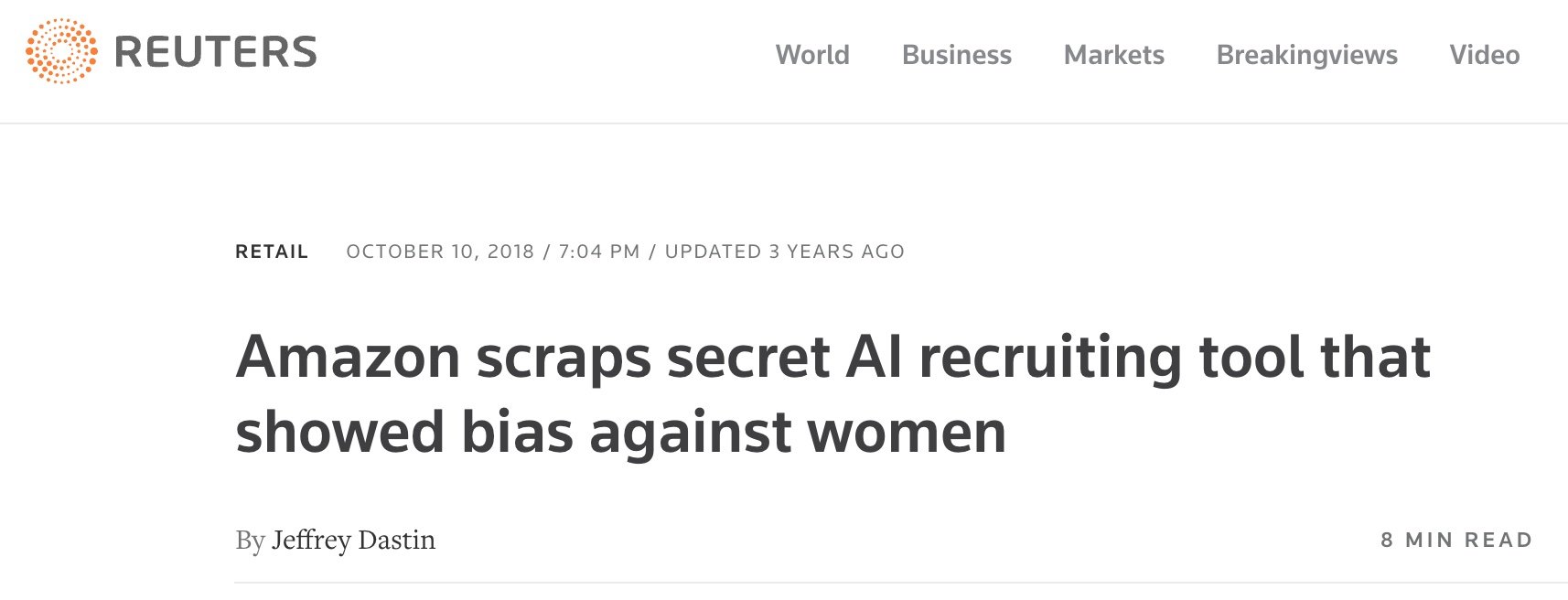
In 2018, machine-learning specialists at Amazon uncovered a big problem: their new recruiting engine did not like women.
The company created 500 computer models to trawl through past candidates' résumés and pick up on about 50,000 key terms. The system would crawl the web to recommend candidates, and then use artificial intelligence to give job candidates scores ranging from one to five stars. It literally gave ratings to people like product reviews on the company’s own storefront.
This was almost certainly due to how the AI combed through predominantly male résumés submitted to Amazon over a 10-year period to accrue data about whom to hire. Consequently, the AI concluded that men were preferable. It reportedly downgraded résumés containing the words "women's" and filtered out candidates who had attended two women-only colleges.
Amazon's engineers tweaked the system to remedy these particular forms of bias but couldn't be sure the AI wouldn't find new ways to unfairly discriminate against candidates. Bias in AI is a very tricky thing to solve, as the models will tend to give answers that predict the training data no matter what.
It gets worse. In the US, some states have begun using risk assessment tools in the criminal justice system. They’re designed to do one thing: take in the details of a defendant’s profile and spit out a recidivism score—a single number estimating the likelihood that he or she will re-offend. A judge then factors that score into a myriad of decisions that can determine what type of rehabilitation services particular defendants should receive, whether they should be held in jail before trial, and how severe their sentences should be. A low score paves the way for a kinder fate. A high score does precisely the opposite.
You probably are already seeing the problem. Modern-day risk assessment tools are driven by algorithms trained on historical crime data, where in the US, blacks have been given harsher sentences.
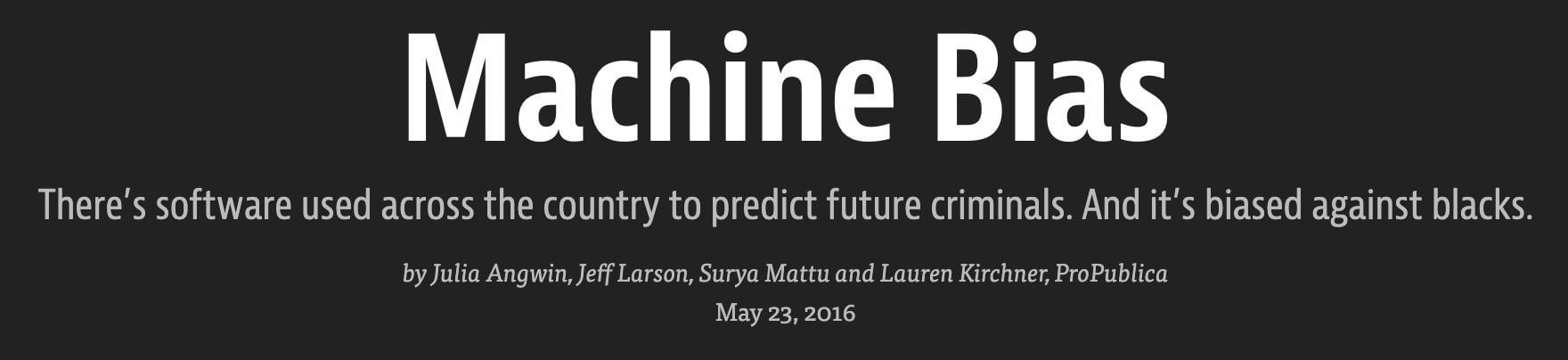
Imprisoning people unfairly, denying employment, and denying everything from insurance to credit cards is known as allocative harm, and it is when a system allocates or withholds certain identity groups an opportunity or a resource.
Structured vs Unstructured Data
Now that we’ve seen how bias in AI is problematic, it begs the question “how can we remove it?”
For some AI, the kind that runs on structured data, this is not that difficult a task. Structured data is data in a table form like a spreadsheet, usually made up of numbers and letters. If we have a table of census data on employment, for example, we could have a spreadsheet with their name, occupation, sex, age, years on the job, and education. In such a situation, it might be an easy step to remove gender bias by just removing the “sex” column from the data.
Even then, this can be problematic depending on the problem being solved. An AI model trained with this data to predict life expectancy might find that the key to a long life is working as a nurse, which could be understood in any number of ways. Perhaps jobs where you actively help people are rewarding enough to make one live longer? Perhaps having close access to medical advice at any time is the key.
The truth, however, is that this would most likely be a simple case of correlation vs causation; in the U.S. approximately 91% of nurses are women, and women live longer than men.
This is the messy, tricky part of working with data. Even supposing somehow the AI could not make mistakes of assuming all nurses are women, it might still become biased in some regard by learning patterns in female and male names. Remove the names, and it might still be biased based on the education data. It’s a challenging problem.
With unstructured data, the challenge grows substantially. Unlike a table where we can directly manipulate the various features, unstructured data such as images, videos, and freeform text is processed by sophisticated large models which can be a bit like a black box. This is an active area of research, and new methods of debiasing models are regularly proposed. Going into detail about how this is done is a bit beyond the scope of this blog post, and we’ll cover it in a future installment.
One thing to be happy about is that models are improving! As more people have become aware of bias in AI, steps have been taken to lessen its presence. Even something as innocuous as emojis on an iPhone has changed in the past few years:
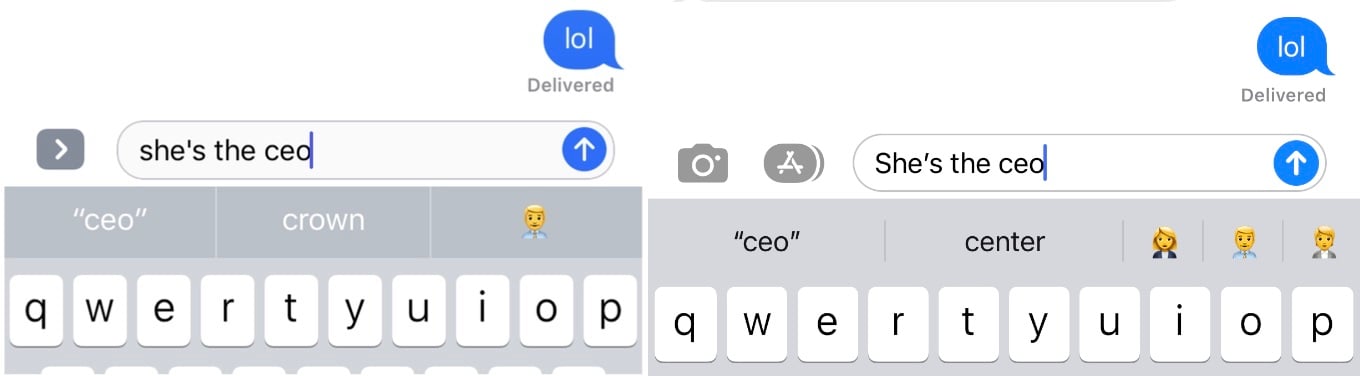
On the left, despite the text indicating the subject of the sentence to be female, the emoji suggests a male only. Today, the term CEO will suggest multiple genders.
Are there downsides to removing bias from AI?
One well-known paper from 2016 is entitled Man is to Computer Programmer as Woman is to Homemaker? Debiasing Word Embeddings. “Word embeddings” are a type of numeric representation of words that machines can understand, and the paper discusses a possible way of removing biases from them.
Interestingly enough, it also addresses one of the philosophical questions of removing bias from AI:
One perspective on bias in word embeddings is that it merely reflects bias in society, and therefore one should attempt to debias society rather than word embeddings. However, by reducing the bias in today’s computer systems (or at least not amplifying the bias), which is increasingly reliant on word embeddings, in a small way debiased word embeddings can hopefully contribute to reducing gender bias in society. At the very least, machine learning should not be used to inadvertently amplify these biases, as we have seen can naturally happen.
This paragraph summarizes two outlooks; one is that we should leave AI models as a reflection of the reality of society, and focus on fixing society’s problems. The second is that because AI models take existing problems and greatly exacerbate them, we should focus on removing them.
My position is that except for certain situations such as the aforementioned life-expectancy predictions, biases should be removed whenever possible. A biased AI can be a racist, sexist monster poisoning society millions of times faster than a human would be able to, and if we do nothing, our models will perpetuate both representational and allocative harms. As well, if we wait for society to be fixed so that our AI models reflect a fair and just society, we’ll be waiting an awfully long time.
Bias occurs when the scope of your training data is too narrow and not diverse enough, but it can also occur due to personal bias. Prejudices can begin to affect a model starting at taxonomy development and onward throughout data curation, which is why Clarifai's Data Strategy team is committed to preventing biases from occurring in models through rigor and an unrelenting commitment to being independent and unbiased in our conduct.
Cuq’oi would be pleased.
Parts of this article, including the definitions of specific harms, the childrens' book, and the emoji example were adapted from a presentation by Ellie Lasater-Guttmann at Harvard University.